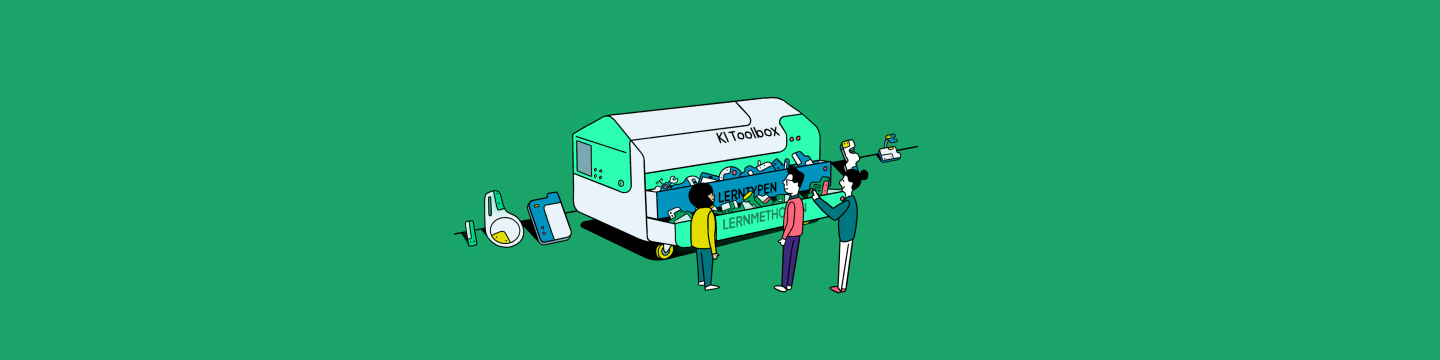
A design framework for creating AI courses in different disciplines
The use of Artificial Intelligence has become key in numerous domains, emphasizing the need for education in this field. The interdisciplinary nature of AI and its relevance across various sectors call for an integration of AI topics into university curricula. In this blog post, Johannes Schleiss introduces the "AI Course Design Planning Framework", a comprehensive tool designed to structure the development of domain-specific AI courses at the university level.
Setting the scene
The increasing importance of AI in today's world is undeniable. AI applications span healthcare, finance, technology, and many more industries, making AI education essential. The call for more AI education is evident in multiple contexts (Laupichler et al., 2022).
Different types of AI education
At the same time, it is important to recognize that there are different types of AI education. In the context of this blog, we distinguish between (1) AI literacy education, providing the foundations to everyone, (2) expert AI education laying the foundation for research and (3) domain-specific AI education offering education at the intersection of AI and domains. While the first two got quite some attention, the latter offers great potential in bringing AI education to different domains (Schleiss et al., 2023).
Domain-specific AI education
There are three reasons domain-specific AI education matters:
- Interdisciplinary Relevance: AI intersects with various fields. Integrating AI education in the domain helps students understand how AI can be applied in their respective domains.
- Enhancing Employability: Graduates well-versed in the application of AI in their domain have a competitive edge in the job market.
- Fostering Innovation: AI education can inspire students to develop AI-driven solutions for real-world challenges.
Challenges in domain-specific AI education
At the same time, domain-specific AI education is challenging for both learners and educators. Learners come from different disciplines and thus bring different prior knowledge and skills. Educators teaching at the intersection of AI and their domain need to bridge two fields. Teaching a domain-specific AI course requires a thorough (self-)reflection of instructors’ competencies in AI and their role in the learning process.
AI Course Design Planning Framework
The following gives a short overview of the categories.
Figure: AI Course Design Planning Framework (Schleiss et al., 2023)
AI in the Domain
- Domain: Discipline where AI is applied, e.g., radiology in medicine or mechanical engineering.
- Use Cases: Identify future potential applications of AI in the domain
- Data in the Domain: Focus on the type of data (e.g. time-series, texts, or images) used in the use cases, considering data types and abundance.
- Implications: Note ethical, legal, and social implications in the use case
- Learning Resources: Identify existing materials that you could build upon, especially Open Educational Resources (OER) that can be integrated
Learning Environment
- Learners: Assess AI skills, clarify roles, and accommodate heterogeneity in interdisciplinary courses.
- Instructors: Assess instructor abilities (e.g. with respect to AI knowledge, domain expertise, and pedagogical skills)
- Internal Support: Recognize resources and limitations, including data, software, and hardware availability.
Course Implementation
- Learning Outcomes: Define specific, measurable objectives aligned with Bloom's Taxonomy.
- Assessment: Balance experiences, connect assessments to real-world applications, and incorporate reflection.
- Learning Activities: Plan diverse learning activities, considering Merrill principles and a mix of teaching methods, including AI-based activities.
How does it work?
The AI Course Design Planning Framework forms a visual and practical tool for instructors and course developers in the higher education or professional education context with a special focus on non-computer science (non-CS) students. It can be used as a means to gather ideas, innovate, plan and communicate ideas for domain-specific AI courses.
The framework can be used as a self-contained instrument for individuals, in tandem with AI and domain experts or in a workshop setting with multiple people. We suggest filling it from left to right, first considering the questions on AI in the domain, the learning environment of the course and last, the course implementation.
Three steps towards better AI education for all
- Try it for yourself
Experimenting is better than reading, so we are excited to have you try it and share your experience. You can download an empty canvas here. - Share the results
We are building up a database of filled frameworks to make it easier for everyone to build AI courses for students. If you want to contribute your filled framework, you can send it to johannes.schleiss@ovgu.de. - Take part in follow-up studies
We are interested in further testing the framework in practice and will evaluate its use in different application areas. Moreover, more workshops around the topic are planned. Currently, we are conducting a study in engineering education. If you are interested in participating, please register here.
References
Laupichler, M. C., Aster, A., Schirch, J., & Raupach, T. (2022). Artificial intelligence literacy in higher and adult education: A scoping literature review. Computers and Education: Artificial Intelligence, 100101.
Schleiss, J., Laupichler, M. C., Raupach, T., & Stober, S. (2023). AI Course Design Planning Framework: Developing Domain-Specific AI Education Courses. Education Sciences, 13(9), 954.