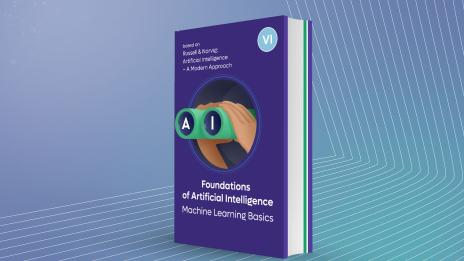
Overview
The course "Foundations of Artificial Intelligence VI" introduces introduces the mathematical foundations of supervised learning and discusses two learning algorithms in depth that are widely used in practice: decision tree learning and neural networks.
Participants learn how to apply these algorithms to practical problems and how to evaluate the quality of a learned classifier. The course concludes with a discussion of the risks and challenges of machine learning.
The course is based on the textbook by Stuart Russell and Peter Norvig: Introduction to Artificial Intelligence, 3rd edition, 2012 or 4th edition, 2020. The 3rd edition is also available in German: Stuart Russell und Peter Norvig: Künstliche Intelligenz - Ein Moderner Ansatz, 3. aktualisierte Auflage, Pearson 2012.
The course is held in English language with German subtitles.
Which topics will be covered?
Module Introduction to Machine Learning
- The learning agent revisited
- Types of learning
- Ockham´s razor
Module Decision Tree Learning
- Boolean decision trees
- Restaurant example
- Overfitting
- Theoretical properties
- Recursive decision tree learning algorithm
- Information Gain and Entropy
- Entropy of a Boolean variable
- Information Gain
- Use in Decision Tree Learning
Module Neural Networks
- Introduction
- History of neural networks
- McCulloch-Pitts “Unit” Model of a Neuron
- Activation functions
- Feed-forward networks
- Single layer feed-forward networks
- Single layer feed-forward networks
- Theoretical properties and limitations
- Backpropagation algorithm
- Take right-turn example
Module Evaluation, Challenges and Risks
- Machine learning workflow
- Training and test data
- Learning curve
- K-fold cross validation
- Evaluating a Boolean classifier
- False Positives and false negatives
- Accuracy, recall, specificity, precision
- F1 Score
- Risks and challenges of machine learning
- Statistical vs. causal models
- Wrong predictions with high confidence
- Universal Adversarial Perturbations
What will I achieve?
By the end of the course, you‘ll be able to
- understand the theoretical foundations of machine learning algorithms,
- evaluate a learned classifier
- apply decision tree learning to practical problems
- apply neural network learning to practical problems
- understand challenges and risks of machine learning algorithms
Which prerequisites do I need to fulfill?
None.
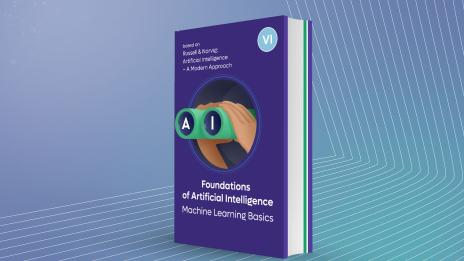
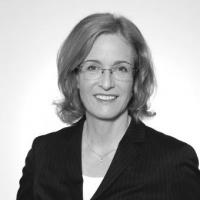
Prof. Dr. Jana Koehler
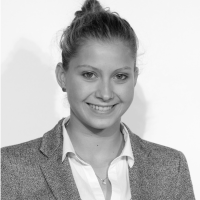
Dr. Sophia Saller
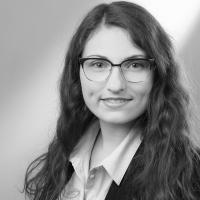